Thoughts from my AGU Turco Lecture
This past December 2020 I had the honor of giving the AGU Future Horizons in Climate Science: Turco Lectureship. If I’m going to be honest, I stressed about this talk for months. How could I possibly contribute anything of substance to such an important and gargantuan topic such as the “future horizons of climate science!?”. It didn’t help that those before me gave fantastic lectures, further adding to my anxiety.
On top of all of that, my research is presently focused on machine learning for scientific discovery — and the thought that I somehow needed to have deep and insightful thoughts about all things machine learning and climate science made me lose sleep at night.
In the end, I decided to stick to what I know, and as it turns out, doing that led me to realize I do, in fact, have opinions about where I think the field should go in this area. Because of this, I want to share a few of them here.
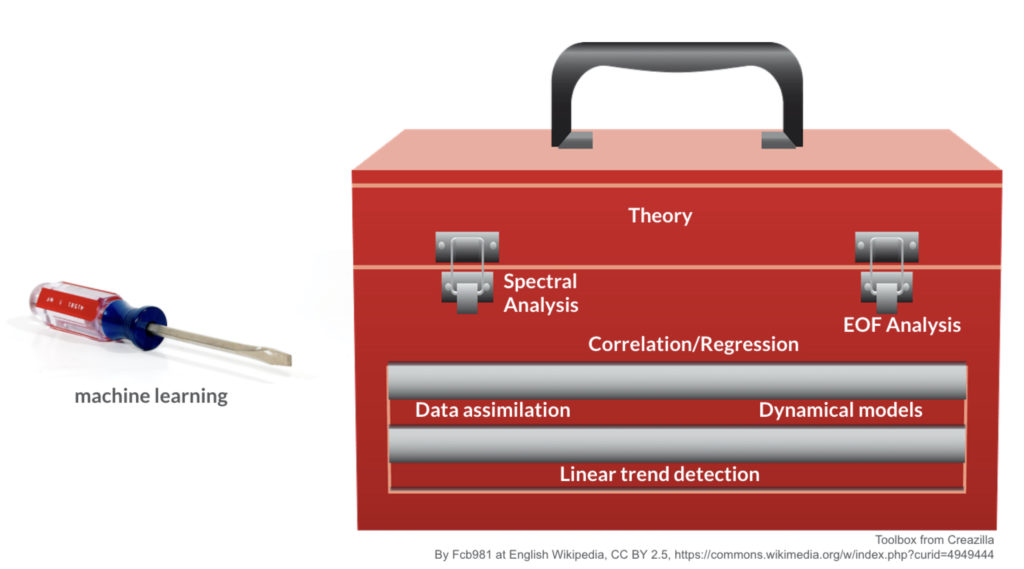
The first and perhaps most important thought, is to acknowledge that the field of climate science already has many methods for data analysis (e.g. data assimilation, regression methods, spectral analysis) and that in my view, machine learning can be viewed as just another tool for the job.
To me, one of our jobs as scientists is to sift through piles of data and try to extract useful relationships that apply elsewhere, i.e. that are applicable “out of sample”. This is what many machine learning methods are designed to do.
As scientists, we are well poised to incorporate machine learning into our research workflow.
While one of many tools, machine learning still has the potential to offer breakthroughs in our field by doing things:
- better
- faster
- cheaper
Just think about how they could transform climate modeling through improving or speeding up convective parameterizations.
With that said, my focus in recent years has decidedly not been on these aspects. Instead, it has been spent exploring how machine learning can teach us something new about the science itself.
We can leverage machine learning to learn new physics!
How might we learn new science from these apparently “black box”, complex models? (I frequently find myself chuckling as I attempt to tune parameters for my neural network – wondering if going out and learning the physical laws of nature through trial and error would in fact be faster). The big idea is that methods now exist to “open the black box” and explain what the neural network has learned. This area of explainable AI, to me, is a game changer for science. It suddenly allows us to ask “why?” and “how?” rather than just make a prediction. And as a scientist, I do love asking “why?”.
Explainable AI has the potential to do many things for machine learning in science, including
1. building trust,
2. ensuring the right answers for the right reasons,
3. assisting users in choosing the right machine learning approach for their problem,
4. (to me, the most interesting) learning new science.
The science can be what the network has learned! We can learn new things by interrogating the machine learning method and asking “how did you get the right answer? What evidence did you use?”. Such an approach let us ask our usual “why?”, but with the power of machine learning.
In my talk I had the opportunity to go through multiple examples of the use of our favorite explainable AI method – layerwise relevance propagation (LRP) – for climate and meteorological applications. So check that out if you are interested. Instead, I would like to leave you with some ideas I have when looking forward to the next 5 years.
Knowledge-Guided Machine Learning The first is the concept of knowledge-guided machine learning. That is, exploring the fusing of scientific knowledge with AI. Why throw out hundreds of years of research into physics, chemistry, etc, just because machine learning is a hot item? How can we blend the two together to get the best of both worlds? This might include incorporating physical laws into the architecture, or using knowledge of the geophysical system to intelligently set up the prediction problem to make the most of explainable AI techniques. Either way, I think this avenue of inquiry is going to be big. And a lot of fun.
Climate Modeling The second and third frontiers revolve around climate modeling. Both leveraging imperfect climate model output through a transfer learning framework, and also improving climate model projections themselves by incorporating machine learning into the building, evaluation and use of climate models. (Of course, an entire blog could be focused on this discussion, rather than one paragraph of one post, so I will just leave this here).
Embracing Data Science Fourth, to truly make the most of these approaches, as a field we must fully accept that data analysis (or data science) is as much a part of our field as fundamental physics. For example, we cannot train our students to be machine learning experts without first teaching them the fundamentals. We need required graduate courses that ensure all atmospheric scientists are well versed in data analysis and statistics.
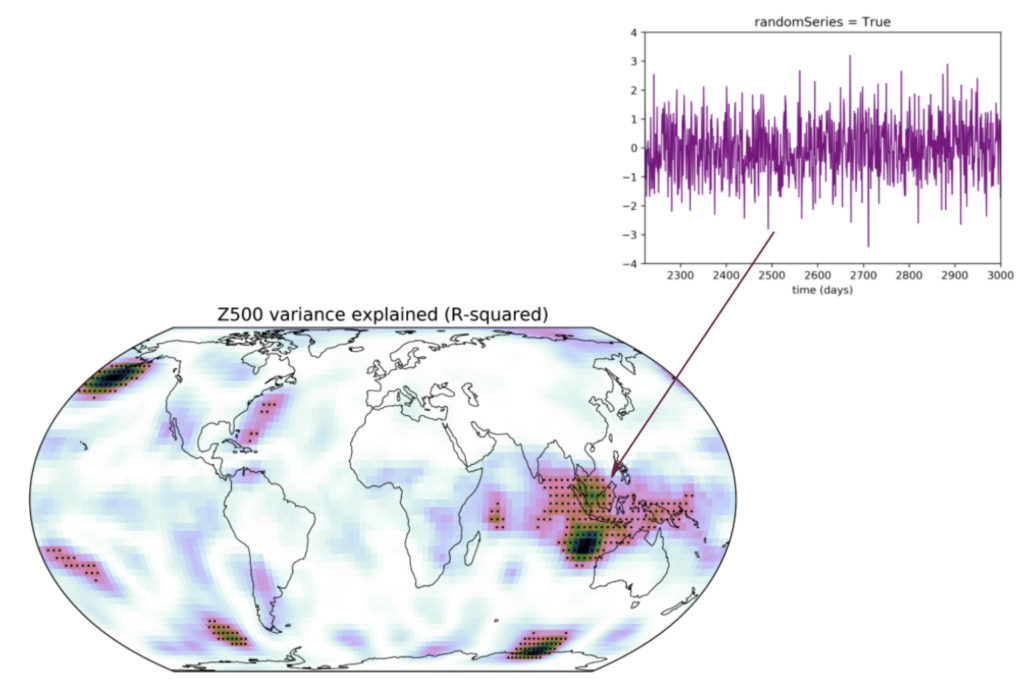
With 40,000 or more grid points on the globe – the possibility of spurious correlation is frightening (see example above of a white noise time series correlated with an atmospheric circulation field). If our students cannot understand this concept, how are they going to interpret and apply the millions of free parameters within neural networks?
In addition, we need to offer and encourage training in data science for all career levels. Yes, graduate students are the future of the field – but let’s not forget that we are all doing science in the machine learning age – and it would be best for everyone to at least vaguely recognize the vocabulary. And finally, we must acknowledge that deriving scientific insights from machine learning methods requires moving beyond off-the-shelf approaches and exploring/developing tools specifically for our field’s needs. This requires a workforce that can think deeply about these topics (and what they need from them) rather than just taking a plug-and-play approach (something made even easier these days with open source software like Keras).
In my mind, our field is well-poised and ready to leverage machine learning for science. For decades, data analysis methods have been an integral part of our field, and we should treat them as such. Machine learning methods are no longer black boxes. Tools exist to help visualize their decisions and this means as scientists we can finally use them to ask “why?”. And I don’t know about you, but that was the reason I got into science in the first place.
For those interested, the full recording of the the 2020 AGU Turco Lecture titled “Explainable AI for the Geosciences” can be found here.